The control engineering take on institutional behavior (2009)
Scope and purpose
The scope of this perspective is intentionally limited to the “Project,” a social system/technical system endeavor defined by the Project Management Institute as “temporary and unique.” Everyone has participated in a great variety of projects involving institutions. Everyone has the background experience to disconfirm the associated mechanisms of action (MoA) derived in this white paper for himself.
The objective here is to define how the power of understanding the MoA, as described in the D4P/D4P4D can be applied by the practitioner to the advantage of an otherwise malfunctioning institution.
Whatever good things we build end up building us. Whatever we build that failed its intended purpose ends up building us. Conclusion? Build. To build, means cooperating with Nature. Control technology is the means by which this collaboration proceeds.
Foundational stipulations
The case for feedforward control
Everyone knows the sorry state of societal affairs in the world today. It is indisputable, in-your-face reality and its various irrational transactions take up a lot of your time. Everyone knows the history of mankind, the endless warfare, the repetitious rise and fall of civilizations and empires.
To the perspective of a control system designer, this historic, global and continuing condition exhibits signature dynamic properties that echo with the knowledge of natural law he incorporates in his process regulators.
The characteristics of social system matters that signal natural law at work include:
- Universal and identical behavior; no exceptions
- Multigenerational; no exceptions
- Extremely resistant to disturbances. No exceptions
- Immortal; the ability of this “Phoenix” to be reborn intact from its own ashes, millennium after millennium. No exceptions.
This behavior set is a classic characterization of a process orchestrated by natural law. It cannot be otherwise. The control designer sees this persisting pattern as a clear imprint of natural law’s handiwork. Such timeless universality of dynamic behavior cannot be attributed to the risk-informed, objectively-reasoned choices of particular hierarchs in the ever-changing cast of players.
The control engineer, sooner or later, cannot help but recognize the fit of what he experiences in engaging projects with social systems, in general, with his knowledge of applied control theory in particular. He does not have to invoke anything outside of his discipline to configure the connection between natural law and institutional (project) behavior using the mathematical physics of control. For this toolbox, all credit goes to Rudolf Starkermann, our mentor.
The extraordinary advantage in investigating institutional behavior equipped with the toolbox of control theory is transparency and the inheritance of its mother, incontrovertibility. Nothing subjective is involved in going from natural law and, riding on control theory alone, connect to human experience. Natural law is deaf to authority and unmoved by credentials. Opinion and professional judgment are peripheral. Empirical evidence plays no role in supporting the concepts. You will find no stories.
Background
We are socially conditioned to believe that while natural law may well apply to animal, vegetable and mineral matter, Homo sapiens are somehow exempt. Because we have exceptional animal intelligence, we are told, we are not bound by the constraints natural law imposes on other, less sapient systems. Thereby, natural law is held insufficient by itself to explain why we incessantly produce the ridiculous history we do.
The fact that no other system can “explain” history with requisite transparency has not budged society from its overwhelming preference for supernatural explanations of nature’s reality, buttressed by cultural folklore proclaimed as infallible by high potentates. This magical thinking is significant for understanding the MoAs of institutional behavior. Once any amount of subjectivity is admitted into the rationale, transparency is lost, reality denial emerges as the behavioral norm.
While it is clear and generally accepted that human history is most certainly the history of its institutions, the prevailing accounts of history (effects) are not scrutably connected to natural law (causes). An animistic view of social system behavior provides nothing for rationalizing a control strategy involving motors, pumps, and valves. Myth going in can only deliver myth2 coming out. As every graduate of Hogwarts has his own myths as to how the world responds to his magic, every application of magic destabilizes the situation. Only one system of universal law can work at a time. Only one.
The arguments herein stipulate that social system behavior is regulated by natural law, and invariant human behavior. The set of laws handed over to run our universe at the big bang have served up universe history for 14 billion years. The rationale herein begins with the statement that natural law is the law of all experience. You will find no stories/case histories used to support the arguments. It’s quite enough to be scrutably connected to natural law. The MoA is transparent and, accordingly, demonstrable – by anyone. Incessant disconfirmation effort is a requisite for maintaining incontrovertibility.
There cannot be an incontrovertible Mechanism of Action (MoA) for explaining the present that fails to account for human history. There cannot be a special MoA that only applies to forecasting the future. Once the natural-law-driven mechanisms-of-action algorithms are validated by benchmarking with reality, like forecasting hurricane tracks, they can be used to go backward and forward in time, like videos. Natural law is a concise way of expressing the connections between reality and the universal force fields that account for experience past, present and future.
The perceptual framework that aligns institutional history must account for social system failures and its successes with the same arguments. It is not one strategy that explains why projects fail and another stratagem that explains why projects succeed. The same natural laws are at work.
The derivation of the MoA for a successful project is covered in detail in the book “Design for Prevention” (D4P ISBN 978-0-937063-05-7). Its Section Seven “Design for Prevention for Dummies” (D4P4D) derives the MoA for social system behavior in an attached book.
This perspective will example how the technology of one discipline, control engineering, connects from its foundation in natural law (control theory) to the MoA of institutional behavior. It will show how control engineering is fully able to transpose its technology to compose the “controller” of social system behavior.
The control theory connection is just one of several independent scrutable pathways through the force fields of natural law that arrive at the same place, an attribute mathematicians call Group Theoretic Isomorphism. So far, four separate logical pathways through the universal force fields have been articulated. This white paper describes only one. Any one is enough.
The history of the application of control theory to social systems is followed by a brief technical description of that part of control theory used to make the metal-flesh connection. Then, an example application of this segment to institutional behavior is presented. Project/institutional services enabled by practitioners who leverage this technology are enumerated.
Start with a reality check
Our thinking in 2009
This most important knowledge for mankind to possess can have no “audience.” The forceful denial of this knowledge is, exactly, how the wisdom it encapsulates grew to be so important in the first place. If an audience existed for this special knowledge, the subject matter of the D4P, the D4P4D and this white paper would have been carved in stone eons ago and human history would have taken a very different course, of course. Because Plan B efficacy is obvious, the lessons learned would have been assimilated into cultural norms many generations before ours. A lot of our ancestors would not have died prematurely in meaningless wars.
Just imagine the challenge to the great designer. You’ve just created Homo sapiens sapiens. It has been deliberately endowed with extraordinary, unprecedented intelligence and reasoning powers. Now that you made intelligence viable, how do you get it to self-destruct, to go extinct by its own hand?
How do you take the intelligent being and selectively blind-spot its intelligence so that the operational reality, in special areas, will be ignored? So that recognition of your nefarious scheme will be suppressed? How do you configure your creation so that the self-destruct syndrome will remain identical generation after generation around the globe at every level of society? That’s quite an order.
Let’s see, give it omnipotent intelligence but embed a self-destruction bent that will survive intact until the species does away with itself. Like the Gadarene swine, just because your social system is in tight formation doesn’t mean it’s on the right track.
How do you get billions of intelligent beings to selectively ignore their own cognition? Where would you begin?
All we do know, via POSIWID and decades of black box testing, is that the great designer succeeded. Its triumph is on display daily. How many times a day do you encounter a situation where boldface facts are being ignored and covered-up to maintain an irrational position? What kind of “intelligence” is that? Can emotional intelligence also be self-destructive?
The practitioner’s dilemma with this master narrative is profound. The obstacle to rationality is not just indifference, but belligerent irrationality. If we don’t reveal the mechanisms of action of institutional project behavior, there’s no way to prevent the endless parade of self-induced calamity. If we do reveal derivation of the MoAs that account for the past, present and future, the cognitively capable audience turns antagonistic. How can the great social system self-destruct impasse be solved if its incontrovertible truth is assigned elephant-in-the-room status?
It is readily demonstrated that Plan B is the pathfinder from doubt to certainty. From reactive to proactive. From instability to stability. From crisis firefighting to prevention. From opaque to transparent. From out-of-control to control. From angst to serenity. From assured failure to assured success – performance, quality and safety. Who wouldn’t want that?
The control engineering platform includes the mechanisms of action of institutional behavior can be understood. Comprehensive testing has confirmed a significant audience for Plan B – one that is intellectually capable of understanding the concepts.
While the interventionist may see the benefits of the D4P concepts as all good, the general population is traumatized by the very same subject matter. What it sees is a likely collision with its installed totem pole of sacred values. Assimilating the truth requires a reallocation of the icons. Adding a totem at the bottom is easy. Displacing the totem at the top is war.
It is difficult to accept that concepts of extreme benefit to intelligent individuals, especially in an unstable society, will be rejected out of hand. No amount of imminent catastrophe facing the social system will be sufficient incentive to place preservation and perpetuation of the social system above those values it actually supports – the ones that encouraged the menace to survival in the first place.
Whatever the superior values are in the minds of the potential audience, it is willing to pay any price to keep them superior. History shows that social systems are more than willing to destroy themselves in a futile attempt to preserve the status quo – POSIWID. Like the Phoenix, the social systems that form out of the ashes and debris of imploded predecessors are acting out the same Greek tragedy. You are surrounded by examples.
In the end it doesn’t matter how much help is available through the D4P concepts. Emotional “intelligence” overrides coherency every time. While there is no entryway for cogent thinking about these matters, that’s how the “audience” wants it. When the price of success demands a context that happens to be in conflict with the cultural norms, failure is always the deliberate choice for (intelligent?) man.
History of the control theory – institutional behavior perspective
Along with the laws of thermodynamics, a key player in the natural law inventory that regulates the movement of the present forward in time, the mechanism of action, is control theory. Looking at unfolding experience as a dynamical system, control theory determines whether the progression of events will be orderly and stable or disorderly and chaotic. The first scientific papers on the direct application of control theory to social system behavior were produced by Rudolf Starkermann starting in 1955. He has written dozens of books presenting his research. William T. Powers published a seminal book, “The Control of Perception,” in 1973 and “Making Sense of Behavior,” in 1998, concerning the application of control theory to individual behavior.
The control engineer designing contraptions to regulate processes is keenly aware of the influence of natural law in general. His virtuosity in mathematical physics and control theory is necessary to decode the dynamics of the process to be controlled. It is the only way to design a fit controller.
In his encounters with reality, the controls design practitioner is like anyone else. With enough exposure, eventually he notices repeating patterns of institutional behavior that his further reflection finds are venerable and universal. He observes that the patterns from ancient time forward are the same and nested. He does not find disconfirming examples at any level of society.
Because of his training in the application of natural laws to his practice, the controls designer is spring loaded to make connections between the controller of industrial process and the “controller” (MoA) of social system behavior. Sooner or later, the Veteran Itinerant Controls Engineer notices that some of the process dynamics he works with in industrial applications have dynamic characteristics strikingly similar to the institutional behaviors he has long observed, studied and endured. His training in natural law forms a perceptual framework that provides an extraordinary view of how human history came to be what it is. He understands that MoAs that decode the past are, exactly, the vehicle for investigating the arriving future. It’s how he designs industrial controllers.
While there are other routes through the natural law inventory besides control theory that end up at the same place, as mentioned earlier, control theory gets there between the covers of the control engineering discipline. It makes no reference to material outside itself and the pathway of logic from theory to observed behavior is direct, coherent and scrutable.
As his experience gathers, curiosity leads the control to prepare dynamic simulations of people systems using the same technology he employs to model the marriage of his control scheme to the technical systems to be regulated. Working the RBF ratchet, he eventually configures a people model that simulates his empirical evidence. VICE then runs the model in regimes he has not yet observed in practice. This is followed up by contriving and running live validation tests in those regimes.
When success with one behavioral set is in hand, innate inquisitiveness leads to repeating the procedure for other behavioral patterns and their ramifications. There is no final all-purpose dynamic model of institutional behavior. There is no final all-purpose model of the disturbance.
Technical elements of the engineering perspective
There are two general categories of controls. One group regulates by hindsight and the other regulates by foresight/anticipation. Applied control theory provides algorithms for both hindsight procedures and foresight procedures. Backward looking regulation, by far the most common sort of control, manipulates a process going forward in time exclusively by the rear view mirror. Even if it was told what was lurking ahead, hindsight control ignores coming attractions until after their fireworks begin. When hindsight control leads, it has no way of using foresight control functionality.
Logically enough, the other category of control is foresight regulation. By looking ahead out the windshield, it synchronizes with the process as both go forward in time. Foresight control always leads but it does include a way for hindsight regulation to contribute.
Hindsight Controls
Most process controllers are of the hindsight class called PID, which stands for proportional, integral and derivative. The control of institutional operations is confined to PID class control because it is vicariously reactive. Institutions are closed-loop systems that decide what action to take solely on the basis of process variable readings – after they change. These controls require no process knowledge – technical system or social system. The setpoint/milestone/benchmark used as reference is guesswork.
In the Proportional control algorithm, the controller output is proportional to the error signal. The error is the difference between the set point (the target reference) and the process variable (instrumentation). This can be mathematically expressed as
When the lag in control response is large, proportional control becomes bang bang (off/on) also known as a hysteresis controller. In control theory, a bang–bang controller is a feedback controller that switches abruptly between two states. Project management is an example of bang bang control.
The contribution from the Integral term is proportional to both the magnitude of the error and the duration of the error. The sum of the instantaneous error over time gives the accumulated offset that should have been corrected previously. The accumulated error is then multiplied by the integral gain ( ) and added to the controller output. It accelerates the movement of the process towards the setpoint and eliminates the residual steady-state error that occurs with a pure proportional controller.
The integral term is given by:
The Derivative of the process error is calculated by determining the slope of the error over time and multiplying this rate of change by the derivative gain .
The derivative term is given by:
The derivative term retards the rate of change of the controller output. It reduces the magnitude of the overshoot produced by the integral component and improves the combined controller-process stability. It slows the transient response of the controller.
The differentiation of an instrument signal is highly sensitive to noise in the error term. The process can become unstable if the noise and the derivative gain are sufficiently large. Starkermann determined the maximum derivative gain, called willpower, that an autonomous individual can exert without going berserk (G=8.0). PID takes feedback signals of process variables as gospel, amplifying any errors in instrumentation it has not been trained to detect.
PID controllers are applicable to many control issues and often perform satisfactorily without any attention or even tuning. The ubiquitous room thermostat is an example.
The fundamental risk with PID control is that it is a hindsight feedback system with constant parameters and no direct knowledge of the process. Control performance is reactive and a compromise.
Without configuring a model of the process, PID control is the best controller you can provide. Advantages include:
- No knowledge of the process, disturbances or the project delivery system is necessary.
- PID control serves the vast majority of industrial process systems quite well.
- PID control technology is proven and standardized. For most applications, software programs are available that will tend to the nagging problem of tuning the gains.
PID is confined by control theory to repetitive process which can tolerate large lags in feedback of error signals. That requisite fits institutional operations seamlessly as long as things are familiar and stable. The control action derived from a comparison of actual to setpoint can only be capricious. The controller has no way to compute in advance the effect of a control action, a change in procedure. This is a characteristic limitation of closed loop control.
- It doesn’t embed a dynamic model of the system, technical or social, being controlled.
- It doesn’t have dynamic models of the disturbance field.
- It doesn’t have a dynamic model (MoA) of the project fulfillment process.
- It doesn’t satisfice Ashby’s Law (D4P)
- It doesn’t satisfice Conant’s Law (D4P)
- It doesn’t support Intelligence Amplification. (D4P)
- The PID controller can drive the process to instability all by itself.
When PID capability fits the process, and most do, things go well. When PID functionality is a misfit to the process to be controlled, PID is a disaster. Since institutions operate exclusively on PID control, the key is the situation. If the institution is rocking along in repetitive production mode, PID control works as well as any.
However, if the institution is being assaulted by novel disturbances proving to be immune to established rule-based task actions, it confuses what has become poison with medicine. With no stop rule, PID magnifies the wreckage being produced with no way to recognize when it is doing so. This is how, exactly, institutional collapse gets to be abrupt.
The chain of command of the institution is a PID controller that circumstances can put way over its head. When hierarchy demands PID control to do what it cannot, the only way to get anything done towards the project goal is to keep the upper echelons out of the loop. If the chain has three or more active command links, control theory sees to it that PID control is counterproductive.
Stability runs amok in hindsight control when there are obstacles and perils ahead that require taking evasive action now. When crisis management is inadequate to the task, prevention is all there is. The lags in hierarchical communication preclude timely intervention.
As mentioned above, control theory supports a different but complementary control scheme that is especially successful with projects and managing large novel disturbances. The scheme achieves control by taking actions that anticipate possible disturbance – actions taken ahead of time that make the disturbance impossible to take effect. “Now” generates the “next.”
Control geared to identify and avert deviations before they would otherwise take place is called feedforward or preventive control. The focus is on human, material and financial resources that flow through a project. It is an intelligence hog.
Feedforward “foresight” controls
Control system design always performs best through a feedforward (or open-loop) controller combined with a PID module for trim. The feed-forward value alone can often provide the major portion of the controller output. Since feed-forward output is not affected by process instrumentation feedback, it lags in responding to large, sudden, novel disturbances. Thereby it can never promote oscillation to instability.
The benefits of feedforward control are significant. Feedforward is stable. Control accuracy can be improved by an order of magnitude. Energy consumption is substantially lower. It reduces wear and tear on equipment (people), lowers (people) maintenance costs, increases reliability and reduces hysteresis.
Feedforward controls require timely, reliable information that is rarely available as a normal part of institutional operations. Providing control-worthy feedforward information requires an effort dedicated to that end. It always has to deal with rooms full of elephants.
The dynamics of a feedforward process controller is a one-for-one fit with the requisites of a successfully-run project.
Since the mechanisms of action are expressed in mathematical physics, the technology provides for a computer-based dynamic simulation facility that can visit the future before it arrives. Equipped with the dynamic model of the MoA, it is easy to project the project trajectory using intelligence amplification
(IA). You can see where the project will go with the procedures it intends to execute.
In feedforward control there is a coupling from the set point and/or from the disturbance directly to the control variable. The control variable adjustment is not error-based. Instead it is based on knowledge about the process in the form of a mathematical model of the process and knowledge about or measurements of the process disturbances.
Feedforward control requires integration of the mathematical model into the control algorithm such that it is used to determine the control actions based on what is known about the state of the system being controlled (Conant’s Law). Its schematic is shown above.
An example of control theory applied to investigate institutional behavior
Initialization
The example selected covers the influence of control theory on the task action choice field available to members of an institution. Using the choice field size available to the autonomous individual as benchmark maximum, there are several natural-law based factors that combine to reduce the span of the choice field actually available to the hierarchy. These include:
1 – Defense of ideological infallibility as it engages the 2nd Law.
Escalating entropy, escalating subreption
Elephants (silence, denial, taboo)
No Front End (D4P)
2 – The Nash Equilibrium (D4P)
Fixed strategy per role
3 – Multiplicity mitigation (D4P)
Ideology in stone
Hierarchical chain of command – # of layers
Ashby’s Law of Necessary Variety
4 – Mismatch Lockdown: operational rigor mortis (D4P4D)
5 – Controller induced instability (the example of this perspective)

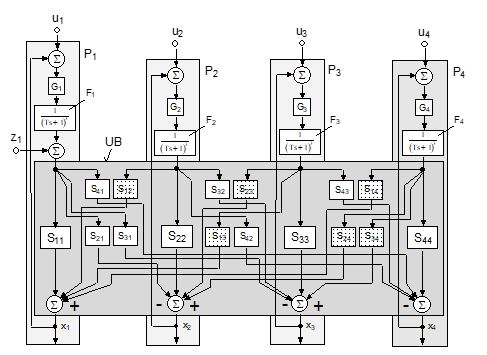
The imminent danger of controller-induced project instability, all by itself, means that practically all of the “choices” made in institutional operations are not reasoned choices at all. System stability is not a function of human volition. No one can make a stable system remain stable through the disturbances attending the arriving future.
The very real threat of instability seriously reduces the management choice field. The five factors automatically integrate to squeeze management options down towards none at all. (the other 4 are derived and described in the indicated companion books) In any institution, the choice field shrinks with time. In a feedforward-controlled project situation, the choice field does not.
In a project, the only possibility to improve/fix things resides at the bottom two tiers – the troops at the work face and their immediate supervisors. A functional disconnect to project productivity occurs at tier 3. It is ironic that the bottom dwellers take the biggest hit in productivity and incentive as more tiers are added to the hierarchy ostensibly to strengthen the infallible chain of command.
The result is that the only place where things project can be made better is hammered into impotency by the hierarchy. Stripped of task action options and any vestige of incentive to reach the goal, the viable levels of the hierarchy bilge shift to robot mode. Management can only bring things to the project that make matters worse.
What no one in the institution suspects is that this sardonic and grossly counterproductive situation is the handiwork of control theory – and nothing else. The players in the drama are powerless to make a beneficial difference. Control theory does nothing to attenuate the choice field for making things worse.
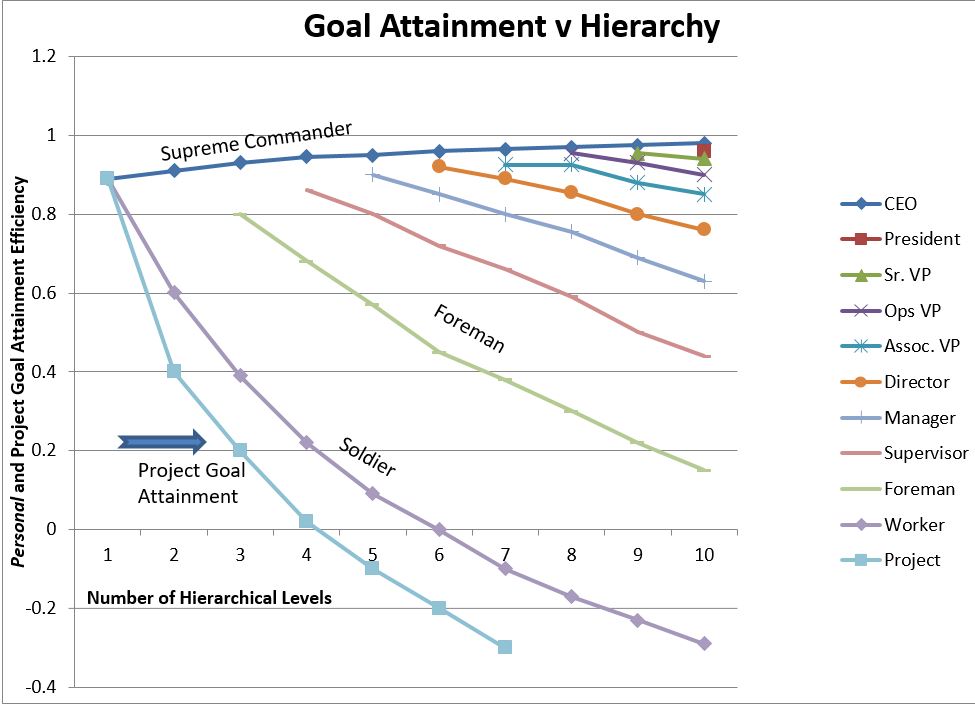
Starkermann’s data on the choice field is the summary of thousands of dynamic simulations performed with a model of social intercourse that has been proven robust for fifty years. His 2010 book on hierarchy summarized the investigation and provided the details. The chart data is from his Hierarchie (in German). The Starkermann schematic of the control theory analog of an individual and the schematic of a four-level hierarchy, shown on the previous page, are taken from his book. As you can see, none of the connected boxes are labeled “professional judgment” or “magic happens here.”
When the data from the simulation runs of the above model configurations started coming out in 2009, even Dr. Starkermann was skeptical of their validity. The simulations were challenging the myth that the chain of command controlled institutional profitability. No humans have been indoctrinated otherwise.
The shared skepticism launched a concerted effort to double check the model and to discredit the results with historical evidence. When no disconfirming examples were found in reality and the model proved coherent, the knowledge was designated incontrovertible. The implications of this investigation are so profound, disconfirmation efforts will continue. Connected to control theory, we see the validity of this pattern everywhere. A chart of the raw data, above, speaks for itself.
Starkermann’s level 1 hierarchy is intended as the autonomous individual. Others may prefer to consider level 1 hierarchy to be the worker and his immediate supervisor. That would make the example 11 levels – what the US Army is today.
Be sure to distinguish Starkermann’s results regarding individual goal attainment apart from his integrations measuring project goal attainment. The only time the two goals coincide is when one is working alone – both supreme commander and soldier in one individual (no hierarchy). As the data attest, the assumption that the top echelon has some control over institutional profitability is a myth. That the executive maintains control over his personal profitability is obvious daily-news fact.
The top tiers choice field gets progressively smaller with both time and number of hierarchical levels up to 4. At and above 5 tiers, the problem-solving choice field available to the head shed is essentially locked on nil. It is important to not confuse choice field size with benefits in prestige and treasure. The top echelons of a tall hierarchy have a miniscule choice field to make things better, but they can choose to make everything else miserable and receive large institutional payouts for their trouble. There is no incentive at the top to tinker with the arrangement. Meanwhile, project Rome burns.
The choice field shrinks with time largely because of the 2nd Law. If the hierarchy has more than 3 levels, it can’t impose enough structure and work to reduce entropy, even if it wanted to. Entropy increase has free reign.
Below 3 levels it is still possible and practical to engage meaningful entropy reduction. The choice field of the autonomous individual remains unlimited in his options to satisfice Ashby’s Law, which is why feedforward control works so well.
The plight of the soldier/squad leader as tiers are added to the hierarchy gets dramatically worse. Going into negative attainment means that the loyal employee is progressively losing ground towards his goal. His obedience to authority drives him away from it. For an example, consider the retirement funding scene today. Corporate management can renege on its retirement plan promise with impunity. The retiree, powerless, has no recourse.
On the way up to the 10-level hierarchy of the chart, the CEO has gained in attainment toward his goal, supreme authority, every tier of the way. This personal benefit comes at the precipitous shrinkage of the ameliorative choice field in his control of the institution. Control choices available to the CEO quickly get to be so limited he is effectively deprived of effective problem-solving capability. He cannot make things better for the institution or the stakeholders. He is not limited at all in his choice field to make matters worse. The venerable adage “The floggings will continue until morale improves” has made that point for centuries.
Be attentive to the CEO’s lament at collapse time and you will hear his ready insistence that he had no power to change the outcome. There is nothing like a US Senate hearing to witness institutional potentates describing, in great detail, their powerlessness to affect outcomes. The choice field/option space shrinks until the residuum contains only bankruptcy or court-ordered liquidation. Thus, the incessant episodes of “sudden” collapse.
As everyone has experienced, goal seeking productivity is closely tied to the available choice field. When the choice field is smaller than the problem field, Ashby’s Law (D4P) kicks in to prevent meaningful progress regardless of the resources applied. Starkermann’s application of control theory develops the case here without reference to any empirical evidence. The natural law basis seals the deal. You can supply the illustrative stories from your own library.
Looking up history and the ongoing actions cannot help but corroborate the algorithms. The most telling validation, always, is the absence of disconfirming examples. Once you have validated the natural law basis by testing, you can’t turn the flood of unsolicited examples off.
The chart above summarizes the investigation findings. The graph plots the size of the choice field available to project participants against the number of levels in the hierarchy. The effect of hierarchy is stunning. The incontrovertible MoA of institutional behavior was constructed entirely from the mathematical physics of control theory. Your task is to disconfirm the claim.
Professor Starkermann comments on his 2009 investigation:
“The model in Hierarchie (ISBN 978-3-905708-58-5) refers to democratic hierarchies. Generally, all elements in a hierarchy are supposed to be treated and arranged equally, i.e., they live ‘socially‘ a democracy and stay under the same laws and rights. But the dynamic simulation runs show that the attainments of the life of elements of a specific hierarchy (in which all n elements are socially equal) are very different and depend on the form of and the kind of information the n elements exchange (consciously and unconsciously) among them.
Even when all people within an organisation are equal, their outcomes (and that is what counts) are far from being equal. From a static standpoint (politically by law) they might be equal, but from the standpoint of life, and life is dynamic, nothing is equal. My n elements of a specific hierarchy initialize with equal power. This is not the case in reality.
Unfortunately our brain is not capable to perceive dynamic behaviour ad hoc. Only symbols can be, or are, equal (as e. g. natural laws are). The outcomes of my models look almost too drastic. All variations in the assumptions of this model, unfortunately, lead to more drastic outcomes.”
Perceptual Control Theory
William T. Powers
How should we go about trying to understand the behavior of people and other living organisms? One way is to look for its causes. This is the approach taken by most scientific psychologists and is the one taught in most courses on research methods in psychology. Using this approach, the causes of behavior are inferred from the results of experiments that look for relationships between independent (stimulus) and dependent (behavioral) variables. If such a relationship is found, and the experiment has been done under properly controlled conditions, then the independent variable can be considered a cause of variations in the dependent variable.
Another way to try to understand behavior is to look for its purposes. This is the approach that people often use to figure out what another person is doing. It involves testing guesses about what the purpose of the behavior might be. For example, a mother is using this method when she tries to understand the crying of her infant by testing her guesses about whether its purpose is to get fed, cleaned, or comforted. This approach to understanding behavior has been largely ignored by scientific psychologists because it assumes that behavior is purposeful.
Purpose has been a problematic concept in the behavioral sciences because it seems to violate the laws of cause and effect. In purposeful behavior cause and effect seem to work backwards in time; a future effect – the result being purposefully produced – seems to be the cause of the present actions that produce it. What we see as purposeful behavior is a process of control, which has a perfectly good scientific explanation in the form of control theory.
Control involves acting to produce intended (purposeful) results in the face of disturbances that would otherwise prevent those results from occurring. Control theory explains how this controlling works. When properly applied to collectives, control theory explains purposeful behavior as control of the perceived results of action. Understanding the purposeful behavior of organisms – the behavior of living control systems — is, therefore, a matter of determining the perceptions that organisms control. Doing research on purpose. There is still much about the technical aspects of doing research on purpose that can only be learned by doing it.
Views: 162